From Data Skeptic to Data Champion - Overcoming Internal Resistance to ML Initiatives
Explore the common reasons behind resistance to Machine Learning projects in organizations and learn practical strategies to build trust, manage change, and demonstrate business value.

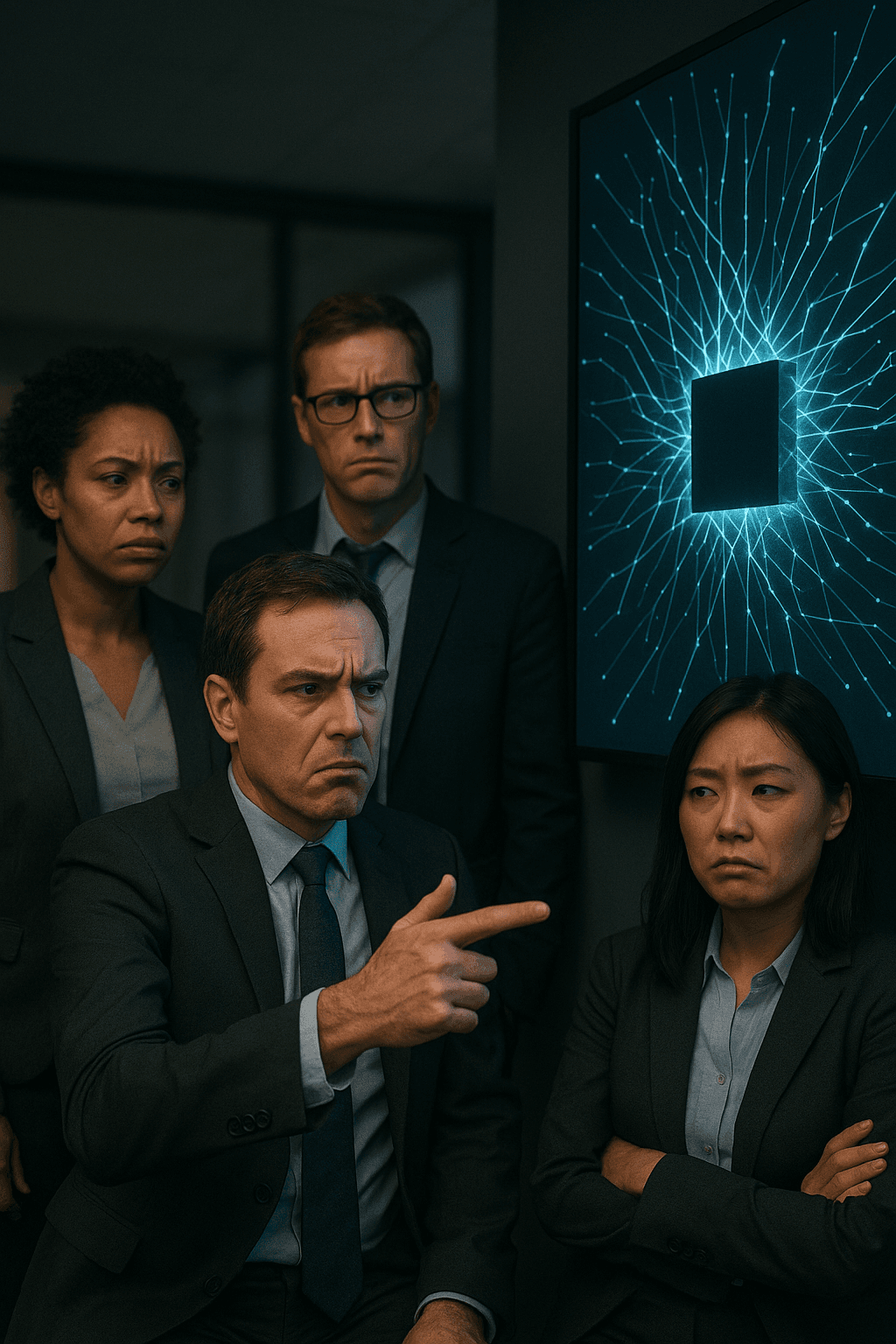
From Data Skeptic to Data Champion: Overcoming Internal Resistance to ML Initiatives
We've all seen the headlines: "AI Revolutionizing Industry," "Machine Learning Drives X% Revenue Growth." It's exciting, it's transformative... but sometimes, within the walls of your own organization, proposing an ML project feels less like sparking a revolution and more like trying to push a boulder uphill.
You've done the research, identified a clear business problem, and built a compelling technical case. Yet, you're met with hesitations, doubts, and sometimes, outright resistance. Why do so many promising industrial ML initiatives face internal skepticism, and how can you bridge the gap between data potential and organizational buy-in?
This isn't just a technical challenge; it's a human and organizational one. The journey from having pockets of data skepticism to fostering a culture of data championship requires understanding the roots of resistance and applying strategic approaches to build trust, manage change, and clearly demonstrate value.
Let's explore how to navigate this critical path.
Section 1: Understanding the Roots of Data Skepticism
Before you can overcome skepticism, you need to understand where it comes from. It's rarely malicious; it's often grounded in valid concerns, past experiences, or a simple lack of understanding. Some common roots include:
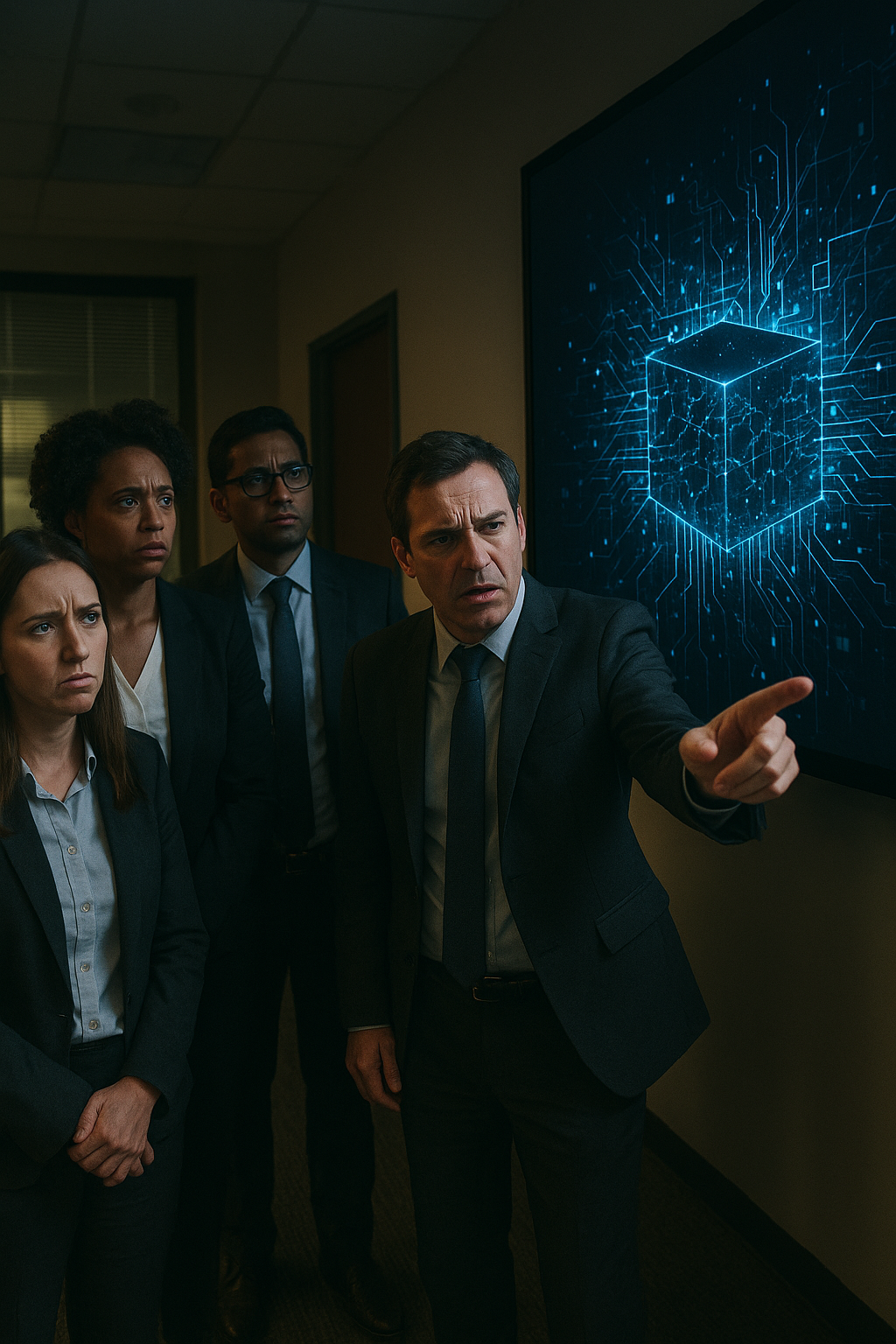
Fig. 1: Skepticism often stems from a lack of understanding or trust in the 'black box' nature of ML.
- The "Black Box" Fear: Machine Learning models, especially complex ones, can feel opaque. Stakeholders who are used to clear, deterministic rules or simple statistical averages may be wary of relying on predictions or decisions generated by something they can't fully "see" or easily explain.
- Data Quality Concerns: Organizations often grapple with inconsistent, incomplete, or inaccurate data. Skeptics rightly question whether fancy algorithms can produce reliable results from what they perceive as "garbage data."
- Fear of Job Security/Change: Automation and data-driven decision-making can be perceived as threats to existing roles or traditional ways of working. Resistance can stem from anxiety about the unknown or the feeling that their expertise is being devalued.
- Past Negative Experiences: Maybe a previous tech project (not necessarily ML) failed to deliver, went over budget, or caused disruption without clear benefit. This can create a general cynicism towards new technological initiatives.
- Lack of Data Literacy: Many business stakeholders simply don't understand the fundamental concepts of data analysis, statistics, machine learning or predictive models. This knowledge gap can lead to mistrust and an inability to evaluate proposals effectively.
- Unrealistic Expectations: Sometimes, skepticism arises from over-hyped promises that ML will magically solve all problems. When initial results aren't instant or perfect, disillusionment sets in.
Recognizing these underlying factors is the first step. It's not about dismissing the skepticism, but acknowledging it and preparing to address it constructively.
Section 2: Building Trust Through Small, High-Impact Wins
You don't win a war against skepticism with a single, massive, enterprise-wide predictive maintenance deployment. You win it with battles – small, strategic victories that demonstrate tangible value and build confidence.
The key here is identifying projects that are:
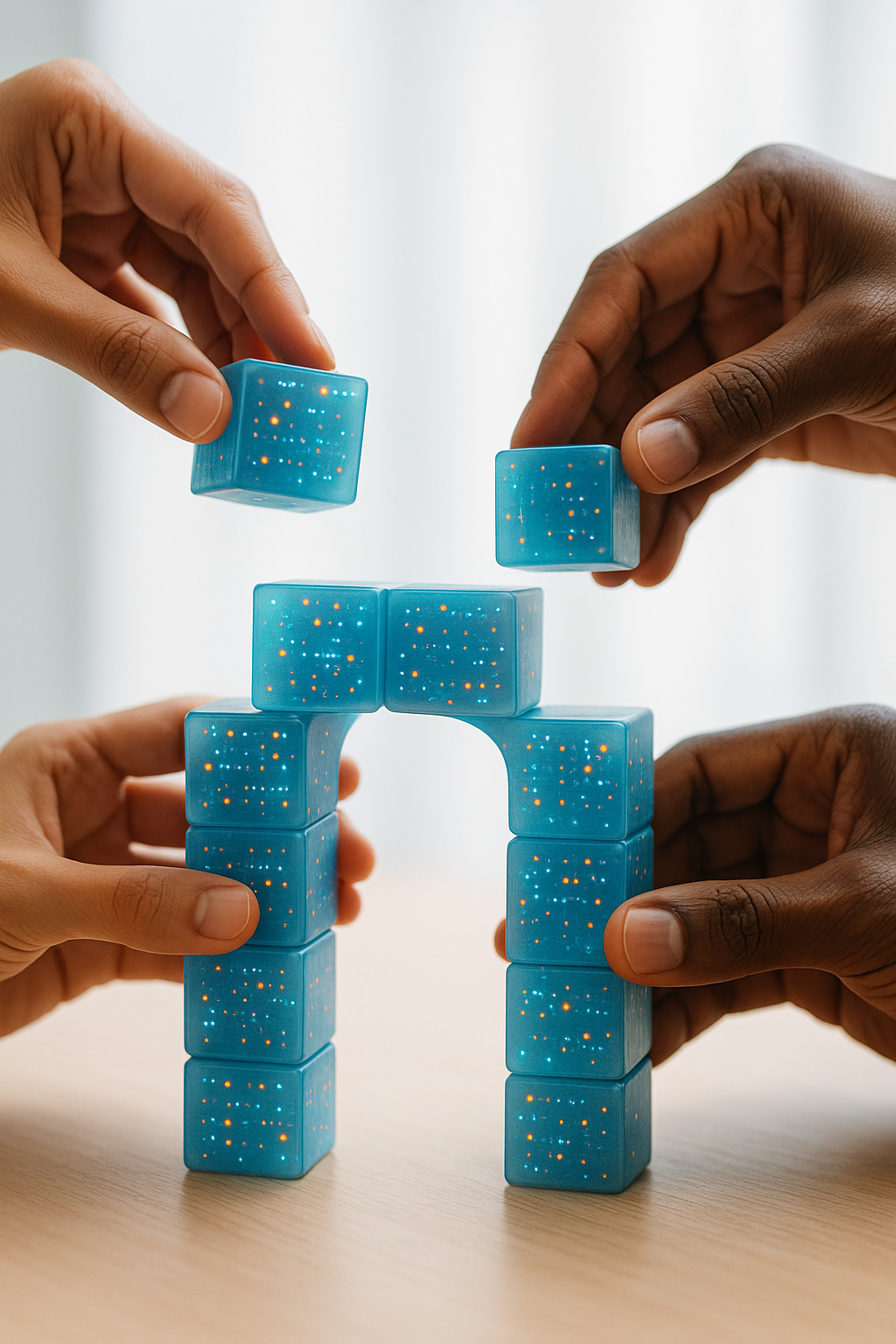
Fig. 2: Building trust step-by-step through small, successful data initiatives.
- Scope-Limited: Avoid sprawling, multi-year initiatives for your first few projects. Focus on a specific problem within a well-defined area.
- Data-Accessible: Choose problems where the necessary data is relatively clean, accessible, and understood. Don't start by tackling a problem that requires Herculean data engineering efforts.
- Visibly Beneficial: Select a problem where a successful ML solution will have a clear, measurable impact that stakeholders can easily understand and appreciate. Think reducing a pain point, saving time, or identifying a clear opportunity.
- Quick to Implement: Aim for projects that can go from concept to demonstrable results (even if it's just a proof-of-concept) within weeks or a few months, not quarters or years.
Example: Instead of trying to predict company-wide customer churn with a complex deep learning model, start with predicting churn for a specific product line using a simpler model and readily available sales data. Demonstrate how identifying even a small percentage of at-risk customers before they leave can lead to targeted interventions and measurable savings.
Each small win acts as a proof point. It provides concrete evidence that ML isn't just hype; it can solve real problems within your organization's context, using your data. These early successes create internal champions – individuals who have seen the value firsthand and can advocate for future initiatives.
Section 3: Strategies for Effective Change Management
Implementing ML is fundamentally about introducing change into how people work and how decisions are made. Successful adoption requires a proactive approach to change management.
- Educate and Demystify: Invest in data literacy programs. Explain core ML concepts in plain language, focusing on intuition and outcomes rather than algorithms. Run workshops, share case studies, and create internal resources. The goal is to make ML less intimidating and more understandable.
- Involve Stakeholders Early and Often: Don't build solutions in a vacuum. Engage the people who will use or be affected by the ML system from the outset. Understand their workflows, pain points, and concerns. Co-creating solutions fosters ownership and reduces resistance. They move from feeling done to to feeling part of the solution.
- Focus on Augmentation, Not Just Automation: Position ML as a tool to assist human experts, not replace them. Highlight how ML can handle repetitive tasks, surface insights, or provide recommendations that empower employees to make better decisions or be more productive. This directly addresses the fear of job displacement.
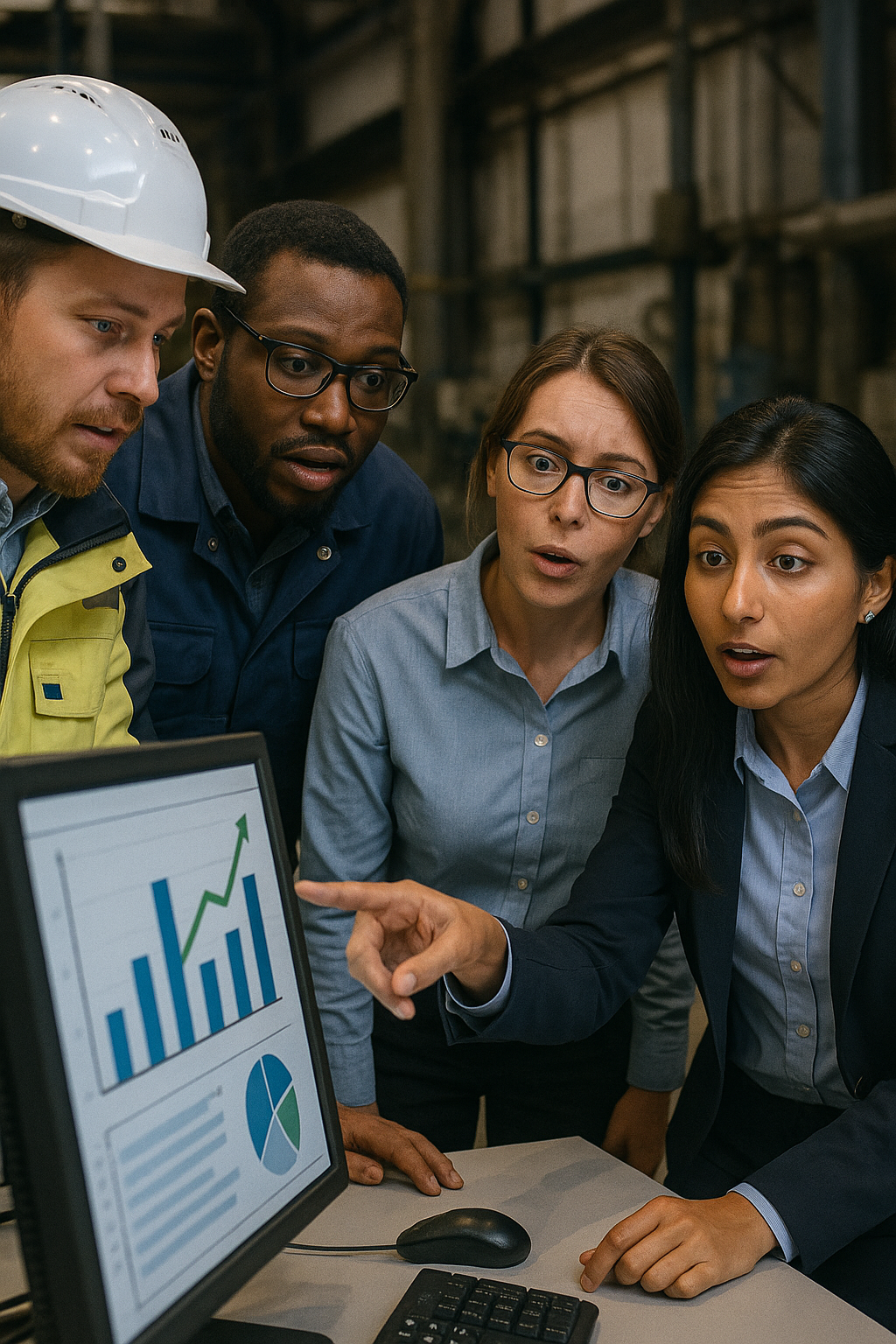
Fig. 3: Involving stakeholders and focusing on collaboration is key to managing change effectively.
- Secure Leadership Sponsorship: High-level buy-in is crucial. Leaders need to visibly champion the data/ML strategy, allocate resources, and communicate its importance across the organization. Their support signals that this is a strategic priority, not just a pet project.
- Create Feedback Loops: Establish clear channels for users and stakeholders to provide feedback on the deployed ML systems. This not only helps improve the models and interfaces but also makes people feel heard and valued, increasing their willingness to adopt the technology.
Change management isn't a one-time event; it's an ongoing process of communication, education, and adaptation.
Section 4: How to Measure and Communicate ML Success in Business Terms
This is perhaps the most critical step in turning skeptics into champions. Data science teams often measure success using technical metrics (accuracy, precision, recall, AUC). While important for model development, these metrics mean little to someone in Sales, Finance, or Operations.
You must translate technical success into business value.
- Identify Relevant Business KPIs: Before starting a project, agree with stakeholders on the key business metrics that will be used to measure success. Examples include:
- Revenue increase (e.g., from personalized recommendations)
- Cost reduction (e.g., from optimized logistics or predictive maintenance)
- Time saved (e.g., automating manual processes)
- Improved efficiency (e.g., faster fraud detection)
- Increased customer satisfaction (e.g., through better service predictions)
- Reduced risk (e.g., identifying potential safety hazards)
- Establish a Baseline: Measure the business KPI before implementing the ML solution. This provides a clear point of comparison.
- Track and Report Impact Consistently: After deployment, continuously monitor the relevant business KPIs.
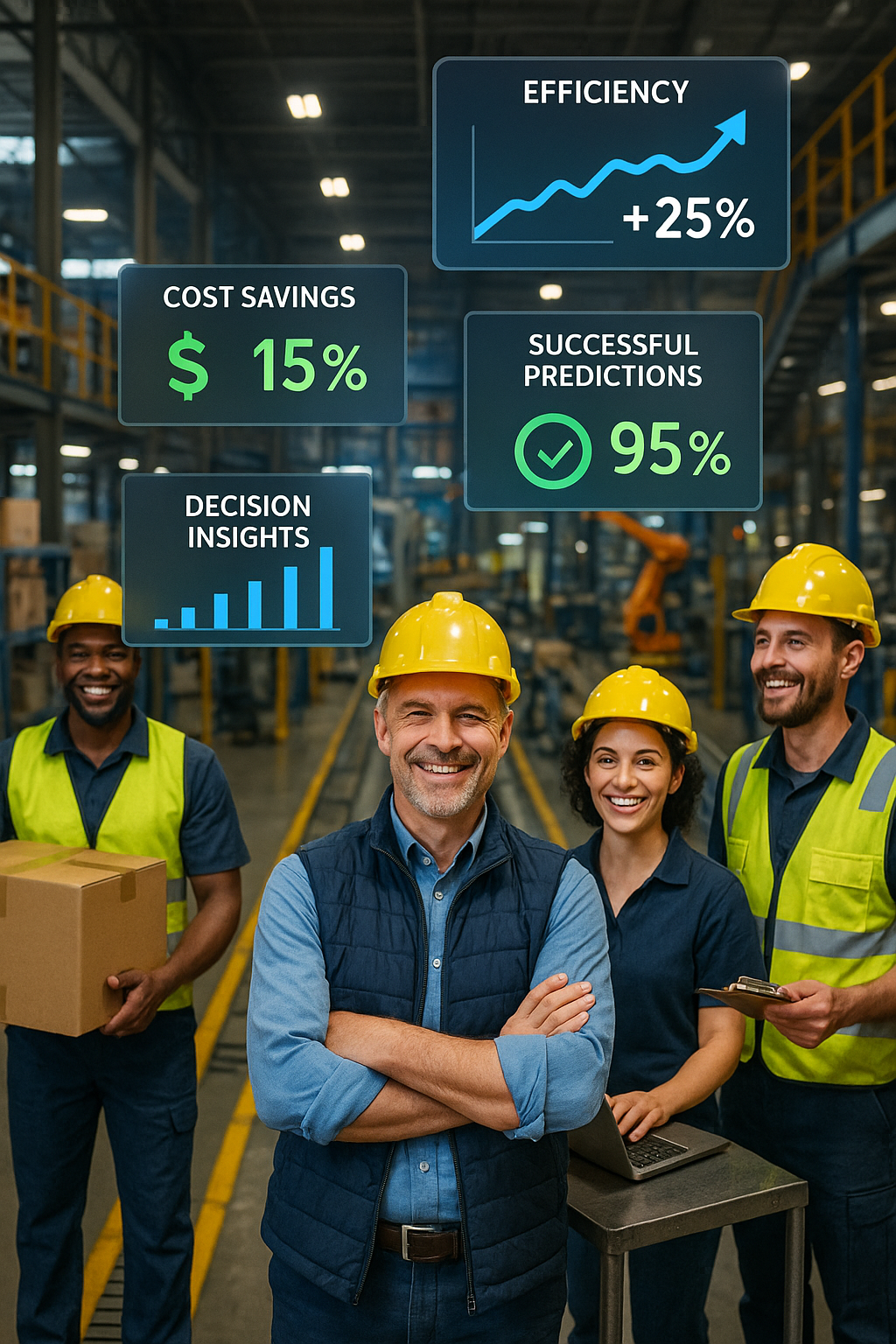
Fig. 4: Communicating ML success using tangible business metrics resonates with stakeholders.
- Communicate Results in a Stakeholder-Centric Way: When presenting results, lead with the business impact. Instead of saying "We achieved 90% accuracy," say "Our model accurately identified potential equipment failures 90% of the time, preventing X hours of downtime and saving an estimated $Y." Use dashboards and reports that visualize the business metrics clearly. Tell a story around the numbers: What problem did you solve, how did ML solve it, and what was the tangible outcome?
Focusing relentlessly on delivering and demonstrating measurable business value is the most powerful antidote to skepticism. It shows that ML isn't just an academic exercise; it's a strategic tool for achieving organizational goals.
Conclusion: Creating a Roadmap for Organizational Data Readiness
The journey from data skepticism to data championship is transformative, but it requires a deliberate, multi-faceted approach. It's not just about hiring data scientists or buying software; it's about cultivating a data-informed culture.
Your roadmap for organizational data readiness should include:
- A Foundation of Data Literacy: Investing in education for all levels.
- A Focus on Strategic, High-Impact Projects: Starting small, demonstrating value, and building momentum.
- Robust Change Management Practices: Involving people, communicating openly, and addressing concerns.
- A Clear Framework for Measuring and Communicating Business Value: Translating technical wins into tangible outcomes.
- Sustained Leadership Commitment: Ensuring data and ML are embedded in the overall business strategy.
By proactively addressing the roots of skepticism, consistently demonstrating value, and championing change, you can transform internal resistance into enthusiastic adoption, turning skeptics into advocates, and ultimately, driving significant impact through Machine Learning initiatives within your organization. The potential is immense – it's time to unlock it, one step at a time.
Related articles
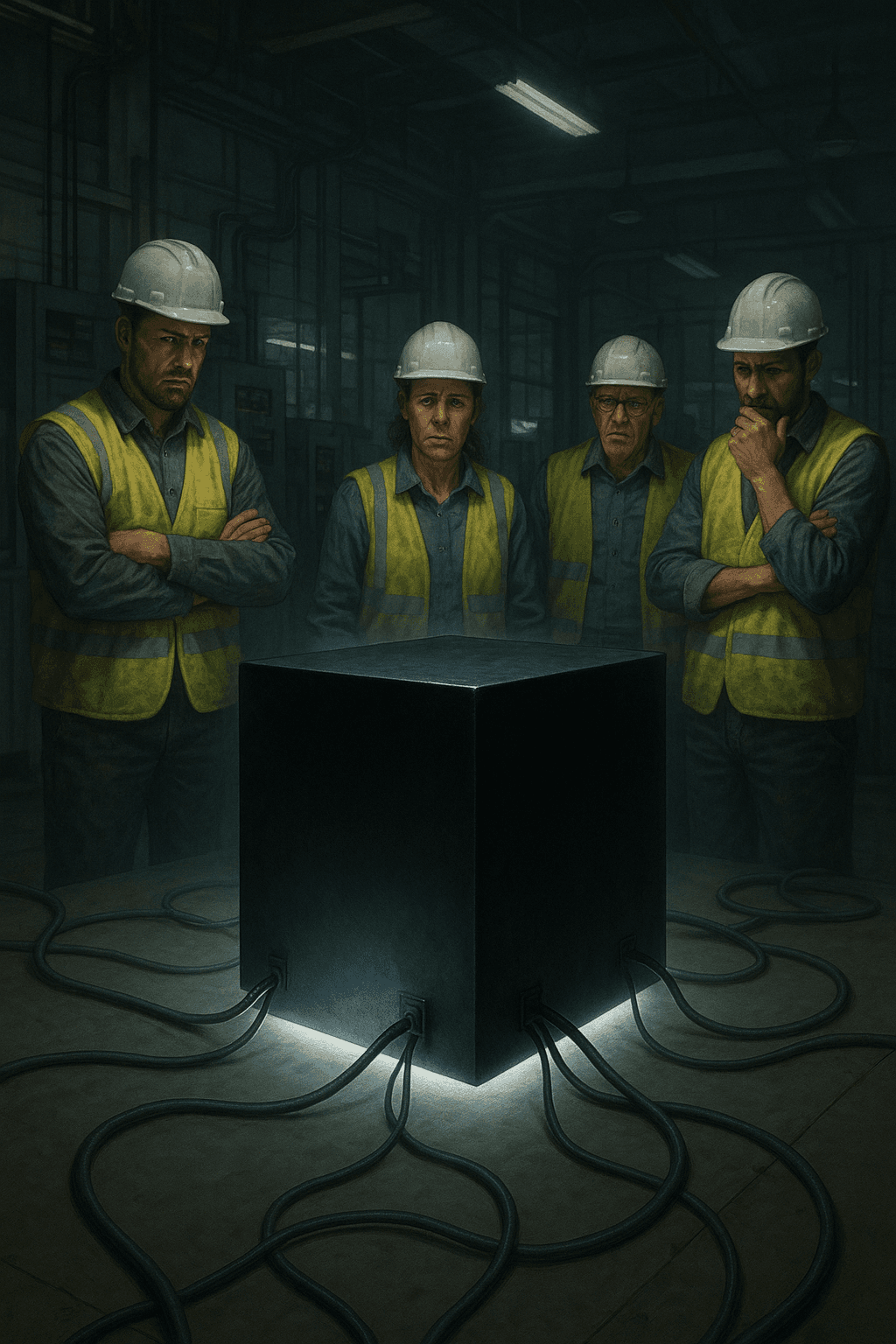
Beyond the Black Box - Making Machine Learning Transparent and Trustworthy in Industry
Discover why explainability is crucial for ML adoption in critical industrial operations and how to build trust through transparency and clear communication.
Ready to get started with ML4Industry?
Discover how our machine learning solutions can help your business decode complex machine data and improve operational efficiency.
Get in touch